Enhancing Home Energy Management: A Day-Ahead Machine Learning Approach Using EMHASS for Predictive Temperature Control
Feb 2, 2025·,·
0 min read
M. Draou
A. Brakez
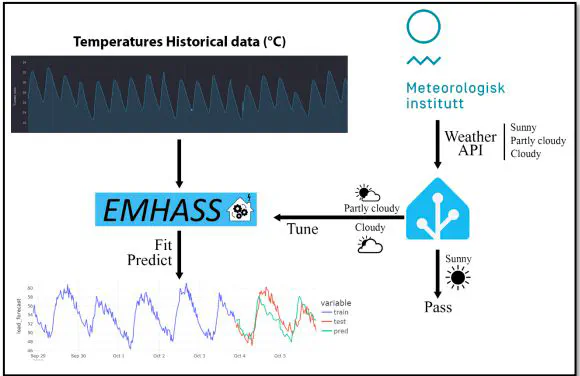
Abstract
This paper presents a comprehensive study on the implementation of a day-ahead machine learning algorithm in residential settings for energy saving purposes. The core of our methodology involves the utilization of the K-Nearest Neighbors (KNN) algorithm for temperature predictions, contributing to the realization of an adaptive and efficient energy management framework. The system collects temperature data at 5-min intervals through temperature sensors, processes it through EMHASS, and trains the KNN model. To address weather-induced variations in thermal energy systems, an automation script checks for foggy conditions, triggering the `tune’ endpoint to adapt the KNN model dynamically. Over the month of November, the model has been evaluated on two renewable energy systems, a hot water system and a space heating system. The findings highlight the effectiveness of our approach in predicting thermal energy system’s temperature with a coefficient of determination R2 evaluated at 91{%} for water heating and 68{%} for space heating. Marking a significant stride in the domain of smart home energy management
Type
Publication
In Trends in Environmental Sustainability and Green Energy